The Ultimate Guide to Data Labeling Platforms: Choosing the Right Tool for Success
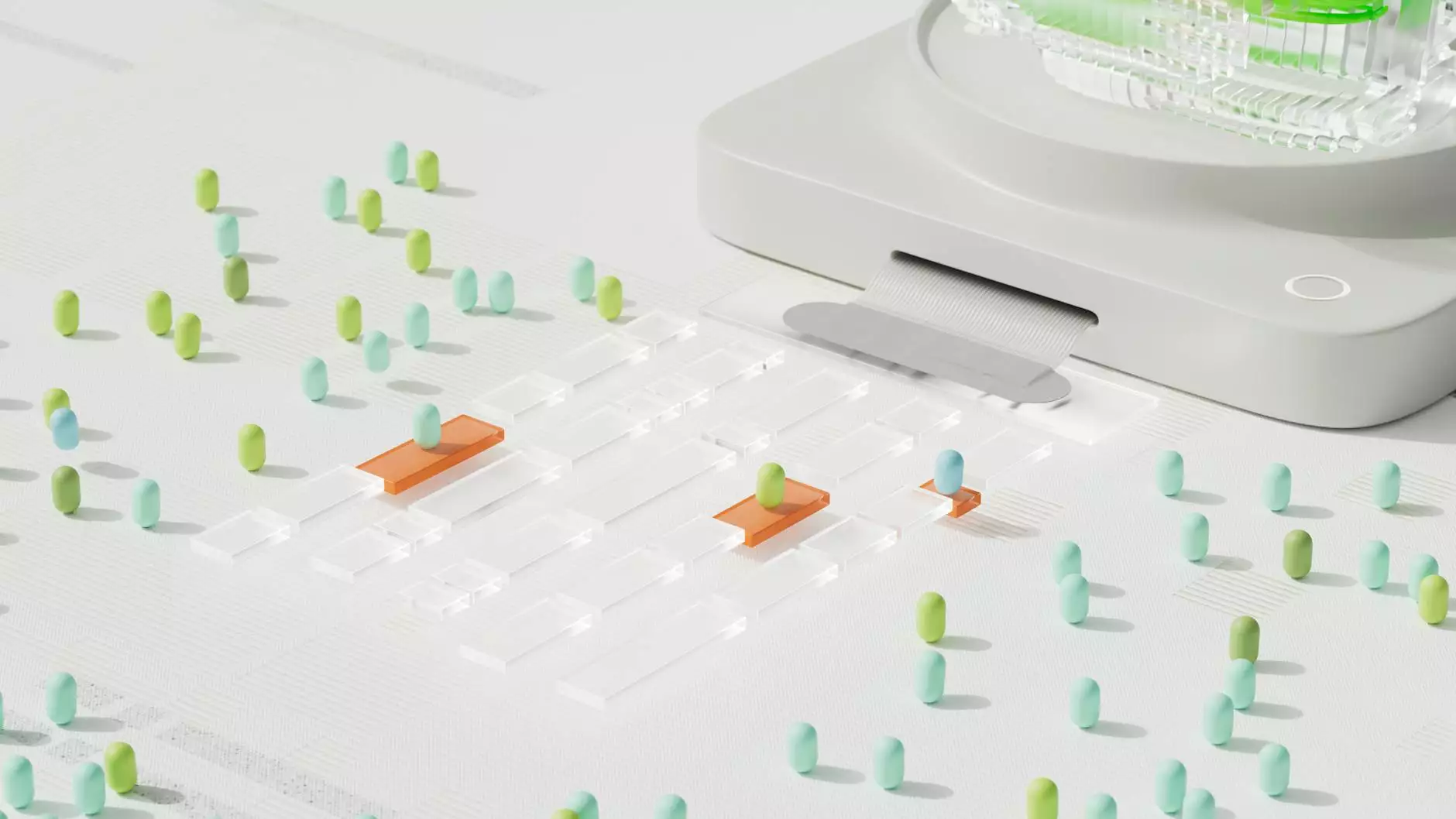
In today's data-driven world, businesses rely heavily on data labeling platforms to enhance their artificial intelligence (AI) capabilities. With technologies advancing at an unprecedented pace, organizations are increasingly seeking resources that offer precise and efficient data annotation. In this comprehensive guide, we will explore the critical elements of data labeling platforms, diving deep into their functionalities and benefits, and why choosing the right platform is imperative for your business's success.
What is a Data Labeling Platform?
A data labeling platform is a specialized tool that allows organizations to annotate datasets, making it easier for machine learning models to learn from them. Data labeling can involve various techniques, including:
- Text Annotation: Tagging or categorizing text data for Natural Language Processing (NLP).
- Image Annotation: Marking up images for computer vision applications, including bounding boxes and segmentation.
- Audio Annotation: Transcribing and labeling audio files for speech recognition systems.
These platforms facilitate the transformation of raw data into a structured format that AI algorithms can effectively utilize, ultimately enhancing the performance and accuracy of AI models.
Why Is Data Annotation Crucial for AI Development?
Data annotation is often regarded as a linchpin in the machine learning process. The accuracy of machine learning models is directly proportional to the quality and volume of labeled data available to them. Some of the key reasons why data annotation is essential include:
- Enhances Model Accuracy: Properly labeled data helps in training models to make accurate predictions.
- Reduces Bias: A diverse and well-annotated dataset minimizes the likelihood of model bias.
- Accelerates Model Training: Efficient labeling processes speed up the model training phase, leading to quicker deployment of AI solutions.
Key Features of an Effective Data Labeling Platform
Choosing the right data labeling platform involves assessing various features that ensure efficiency, effectiveness, and scalability. Here are some essential attributes to consider:
1. User-Friendly Interface
Intuitive design and usability are crucial for data labeling platforms. A user-friendly interface allows teams to navigate the platform efficiently, reducing the learning curve associated with new tools.
2. Scalability
As your data needs grow, so should your platform. The ability to scale easily ensures that you can handle increasing volumes of data without compromising quality.
3. Multi-format Support
A competent data labeling platform supports various data types (images, videos, text, and audio), enabling a holistic approach to data annotation across different projects.
4. Collaboration Features
In a corporate setting, diverse teams often work together on data annotation projects. Collaborative tools that allow multiple users to annotate data simultaneously can significantly enhance productivity.
5. Quality Assurance Tools
To maintain high-quality standards, platforms should include features for quality control, such as review mechanisms and feedback loops, ensuring that annotations are accurate and reliable.
Types of Data Annotation Techniques
Data labeling encompasses a variety of annotation techniques, each suited for different applications. Understanding these techniques is vital for selecting the appropriate data labeling platform. Common techniques include:
1. Image Annotation
This involves marking specific features within images, often through bounding boxes, polygons, or segmentation. It's widely used in training computer vision models for applications like:
- Object Detection
- Image Classification
- Facial Recognition
2. Text Annotation
Text annotation involves tagging parts of speech, sentiment, and other relevant attributes in textual data. It is crucial for NLP tasks such as:
- Chatbots and Virtual Assistants
- Text Classification
- Sentiment Analysis
3. Audio Annotation
This process includes transcribing audio data, labeling speaker identities, and marking different audio events, which is essential for systems like:
- Speech Recognition
- Audio Event Detection
- Voice Analysis
The Role of Keylabs.ai in Data Annotation
When it comes to data annotation tools, Keylabs.ai stands out as a premier platform that combines the most essential features needed for effective data labeling. Here’s what makes Keylabs.ai a preferred choice:
1. Cutting-Edge Technology
Keylabs.ai leverages advanced artificial intelligence and machine learning algorithms to enhance the data annotation process. With machine-assisted labeling, you can achieve quicker results without sacrificing quality.
2. Robust Annotation Toolset
The platform provides a wide range of annotation tools for various data types, ensuring that teams have the flexibility they need to cater to specific project requirements. Whether you need image segmentation or audio labeling, Keylabs.ai has you covered.
3. API Accessibility
For organizations looking for seamless integration, Keylabs.ai offers APIs that allow businesses to connect their data sources with the annotation platform effortlessly.
4. Customized Workflow
Different projects come with unique needs. Keylabs.ai enables users to customize workflows that best fit their annotation processes, leading to better resource allocation and enhanced productivity.
How to Choose the Right Data Labeling Platform
Considering the myriad of options available, selecting the right data labeling platform can be overwhelming. Here are some strategic steps to guide your decision-making process:
1. Assess Your Needs
Prior to choosing a platform, it is vital to evaluate your organization's specific needs, including data volume, data types, and budget constraints. Understanding the scope of your project will help in selecting a suitable platform.
2. Test Features
Many platforms offer free trials or demos. Utilize these opportunities to test the features of different platforms and observe how they align with your requirements.
3. Check for User Reviews and Testimonials
Research user feedback and experiences with different platforms. This will provide insights into the reliability and performance of the platforms you're considering.
4. Evaluate Customer Support
Effective customer support is paramount, especially during implementation and initial usage. Ensure that the platform offers responsive support through multiple channels.
5. Data Security and Compliance
With concerns over data privacy on the rise, it is crucial to choose a platform that adheres to stringent security protocols and industry regulations. Look for features that ensure your data remains safe and secure during the annotation process.
Conclusion
In summary, navigating the landscape of data labeling platforms requires careful consideration of various factors, including functionality, usability, and specific project needs. Platforms like Keylabs.ai exemplify the qualities needed for effective data annotation, providing businesses with the tools to enhance their AI capabilities and drive innovation. By investing in an appropriate data labeling platform, organizations position themselves to thrive in an increasingly competitive, data-driven world.
As we move forward into the future of AI and machine learning, remember that the importance of quality data annotation cannot be overstated. Embrace the tools available, and harness the power of data to unlock potential and propel your business to new heights.